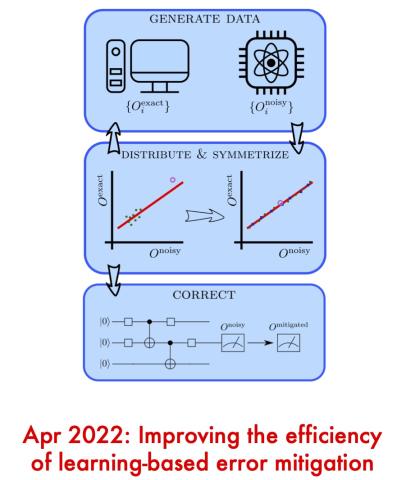
Error mitigation will play an important role in practical applications of near-term noisy quantum computers. Current error mitigation methods typically concentrate on correction quality at the expense of frugality (as measured by the number of additional calls to quantum hardware).
To fill the need for highly accurate, yet inexpensive techniques, we introduce an error mitigation scheme that builds on Clifford data regression (CDR). The scheme improves the frugality by carefully choosing the training data and exploiting the symmetries of the problem.
We test our approach by correcting long range correlators of the ground state of XY Hamiltonian on IBM Toronto quantum computer. We find that our method is an order of magnitude cheaper while maintaining the same accuracy as the original CDR approach.
For details see
“Improving the efficiency of learning-based error mitigation”, Piotr Czarnik, Michael McKerns, Andrew T. Sornborger, Lukasz Cincio. arXiv:2204.07109.