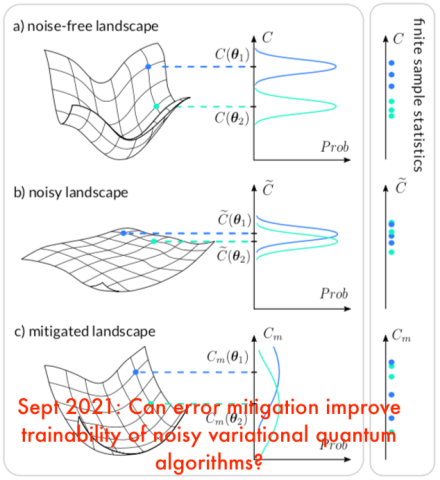
Variational quantum algorithms (VQAs) may be the best hope for near-term quantum advantage, but noise significantly affects their performance. We study whether error mitigation can improve the trainability of VQAs. We find that error mitigation cannot solve the exponential scaling associated with noise-induced barren plateaus, but it can improve trainability in some cases.
Our work implies that noise-induced barren plateaus are a serious issue that cannot be simply solved with error mitigation. On the other hand, we find that one could tailor the error mitigation method to somewhat improve trainability, and hence our work highlights the need to develop error mitigation methods that help in this regard.
For details see
“Can error mitigation improve trainability of noisy variational quantum algorithms?”, S. Wang, P. Czarnik, A. Arrasmith, M. Cerezo, L. Cincio, P. J. Coles. arXiv:2109.01051.